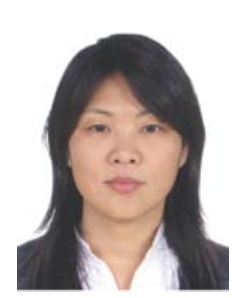
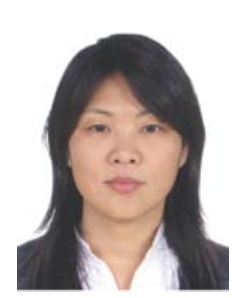
水及离子水合物的表面性质在生物、化学、能源、材料等多个领域都发挥了重要作用,氢键网络的复杂性与动力学性质、质子传输机制以及水冰预融现象紧密相关。要想理解水的表面行为,了解离子在附着于衬底时如何进行排列,就需要对水内部的氢键网络结构进行深入研究。目前,通过密度泛函(DFT)计算和原子力显微镜(AFM)图像模拟等手段能够一定程度上推测出氢键网络的结构及其形成过程,同时通过机器学习等方法缩减AFM图像模拟过程所消耗的时间,从而实现对水和离子水合物表面行为的微观机理解释,并通过对这一机制的理解将水的表面特性实现更为广泛的应用。
代表性文献:
1. Y. Tian*, J. Hong*, D. Cao*, S. You*, Y. Song, et al. "Visualizing Eigen/Zundel cations and their interconversion in monolayer water on metal surfaces", Science 377, 315-319 (2022).
2. B. Tang*, Y. Song*, M. Qin*, et al. "Machine learning aided atomic structure identification of interfacial ionic hydrates from AFM images", National Science Review. nwac282 (2022).
3. Cao, DY, Song, YZ, Peng, JB, et al. "Advances in Atomic Force Microscopy: Imaging of Two- and Three-Dimensional Interfacial Water", Front Chem. 9, 745446 (2021)
4. Cao, DY, Song, YZ, Peng, JB, et al. "Advances in Atomic Force Microscopy: Weakly Perturbative Imaging of the Interfacial Water", Front Chem. 7, 626 (2019)
5. J. Peng, D. Cao, Z. He, et al. "The effect of hydration number on the interfacial transport of sodium ions", Nature 557, 701-705 (2018).